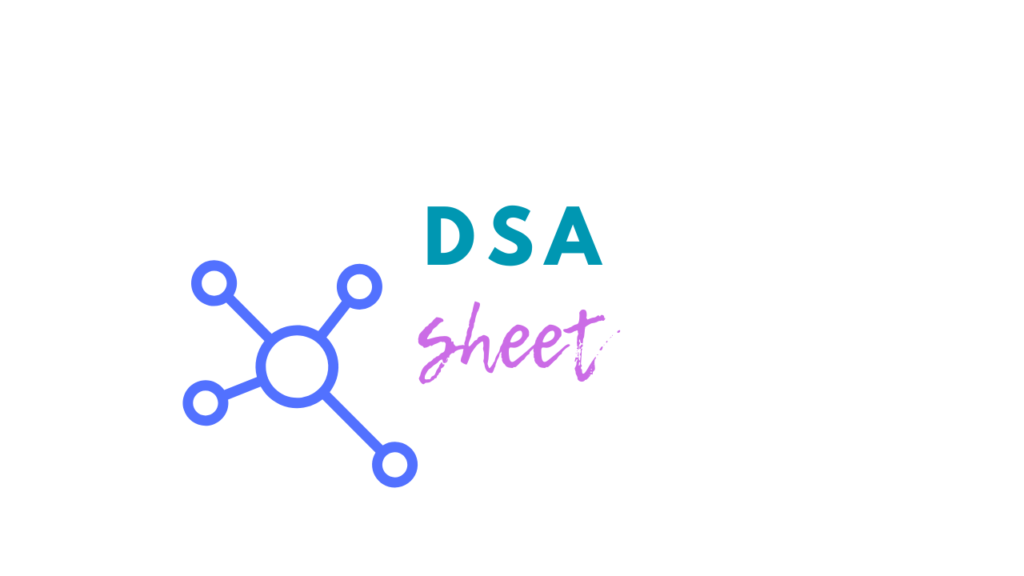
Problem Statement (Asked By Amazon)
Given an integer k
and a string s
, write a function to determine the length of the longest substring in s
that contains at most k
distinct characters.
For example, given s = “abcba” and k = 2, the longest substring with k distinct characters is “bcb”.
Problem link: https://leetcode.com/problems/longest-substring-with-at-least-k-repeating-characters/
Disclaimer: Try solving the problem on your own first! Use this solution only as a reference to learn and improve.
The brute force approach is to generate every possible substring and check if it contains at most k distinct characters. If it does and its length is greater than the current longest valid substring, we update the longest substring. This approach is inefficient as it requires O(n^2 x k) time — O(n^2) to generate substrings and O(k) to check distinct characters.
Brute Force Solution O(n^2 x k):
import java.util.HashSet; public class LongestSubstringKDistinct { public static int longestSubstringWithKDistinct(String s, int k) { if (k == 0 || s == null || s.isEmpty()) { return 0; } int maxLength = 0; for (int i = 0; i < s.length(); i++) { for (int j = i + 1; j <= s.length(); j++) { String substring = s.substring(i, j); if (countDistinctCharacters(substring) <= k) { maxLength = Math.max(maxLength, substring.length()); } } } return maxLength; } private static int countDistinctCharacters(String s) { HashSet<Character> distinctChars = new HashSet<>(); for (char c : s.toCharArray()) { distinctChars.add(c); } return distinctChars.size(); } public static void main(String[] args) { System.out.println(longestSubstringWithKDistinct("abcba", 2)); // Output: 3 } }
Optimized Sliding Window Solution O(n×k):
A more efficient approach uses the sliding window technique:
- Maintain a dictionary to track the last occurrence index of each character within the window.
- Expand the window by adding characters to the dictionary.
- If the size of the dictionary exceeds k, shrink the window by removing the least recently used character (based on the smallest index).
- Update the maximum length of the substring at each step.
import java.util.HashMap; public class LongestSubstringKDistinct { public static int longestSubstringWithKDistinct(String s, int k) { if (k == 0 || s == null || s.isEmpty()) { return 0; } HashMap<Character, Integer> lastSeen = new HashMap<>(); int maxLength = 0; int left = 0; for (int right = 0; right < s.length(); right++) { char currentChar = s.charAt(right); lastSeen.put(currentChar, right); // If the window size exceeds k, shrink it if (lastSeen.size() > k) { int leftMostIndex = Integer.MAX_VALUE; char charToRemove = '\0'; for (char c : lastSeen.keySet()) { if (lastSeen.get(c) < leftMostIndex) { leftMostIndex = lastSeen.get(c); charToRemove = c; } } lastSeen.remove(charToRemove); left = leftMostIndex + 1; } // Update maximum length maxLength = Math.max(maxLength, right - left + 1); } return maxLength; } public static void main(String[] args) { System.out.println(longestSubstringWithKDistinct("abcba", 2)); // Output: 3 } }
Explanation of Sliding Window:
- Tracking Characters:
- Use a
HashMap
to track the last occurrence index of each character in the current window.
- Use a
- Expanding Window:
- As you iterate through the string, add the current character to the map and update its index.
- Shrinking Window:
- If the size of the map exceeds k, identify the character with the smallest index (the least recently used character) and remove it. Adjust the left pointer of the window accordingly.
- Updating Result:
- After each step, calculate the length of the current window and update the maximum length.
Complexity:
- Time Complexity:
- O(n×k), where n is the length of the string and k is the number of distinct characters (due to the
HashMap
operations).
- O(n×k), where n is the length of the string and k is the number of distinct characters (due to the
- Space Complexity:
- O(k), as the
HashMap
stores up to k characters.
- O(k), as the
This approach is efficient and works well for large strings with a reasonable value of k.
Did this solution help you understand the concept better? Let me know your thoughts in the comments below! Don’t forget to follow us on Instagram @coderz.py for more DSA tips and solutions to ace your coding interviews.
Leave a Comment
You must be logged in to post a comment.